When the model resorts to unfaithful reasoning
This week: Biology of a LLM, entrepreneurs as theorists, a grammar of hypotheses
On the Biology of a Large Language Model
Is bullshitting conflated with insisting on trying even when it’s too hard?
“Our methodology is intended to expose the intermediate steps a model uses en route to producing a response.”
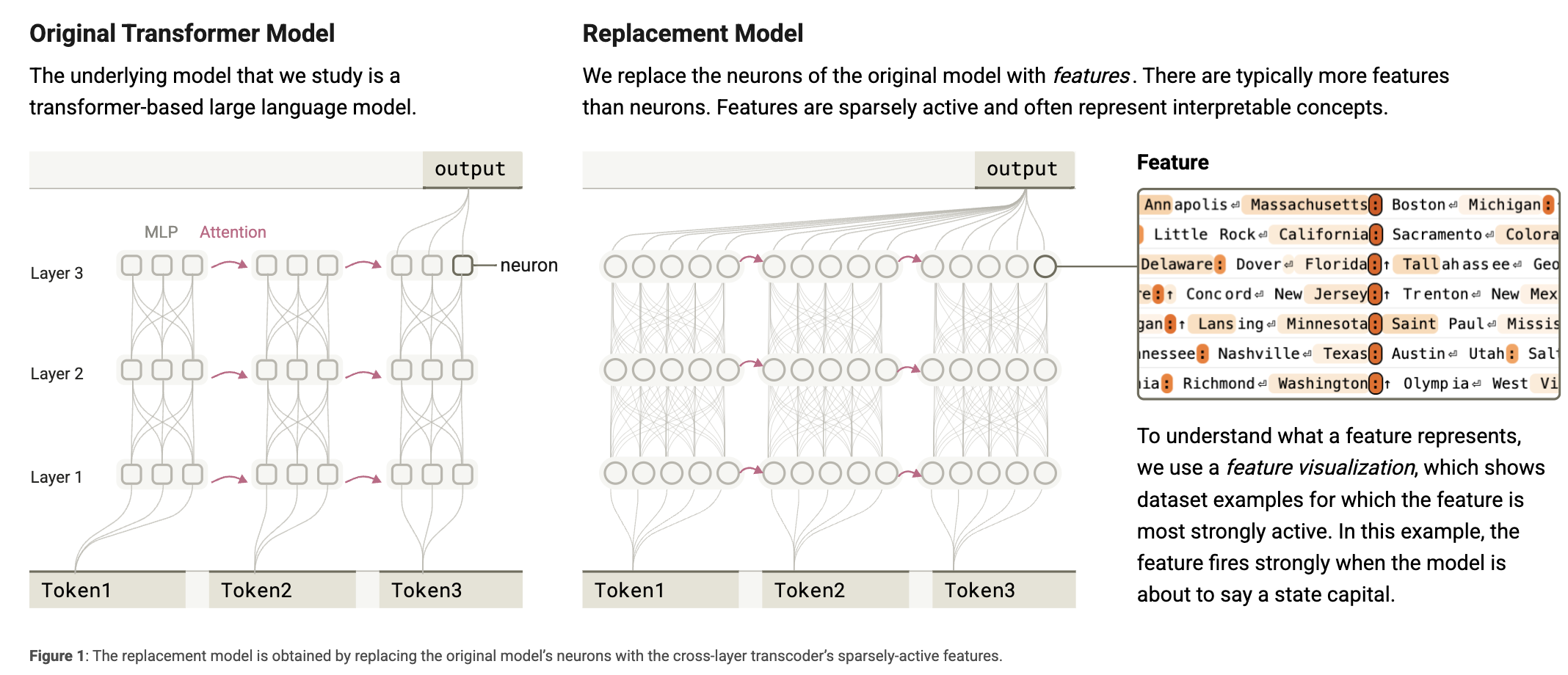
“Progress in AI is birthing a new kind of intelligence, reminiscent of our own in some ways but entirely alien in others. Understanding the nature of this intelligence is a profound scientific challenge, which has the potential to reshape our conception of what it means to think. The stakes of this scientific endeavor are high; as AI models exert increasing influence on how we live and work, we must understand them well enough to ensure their impact is positive. We believe that our results here, and the trajectory of progress they are built on, are exciting evidence that we can rise to meet this challenge.”
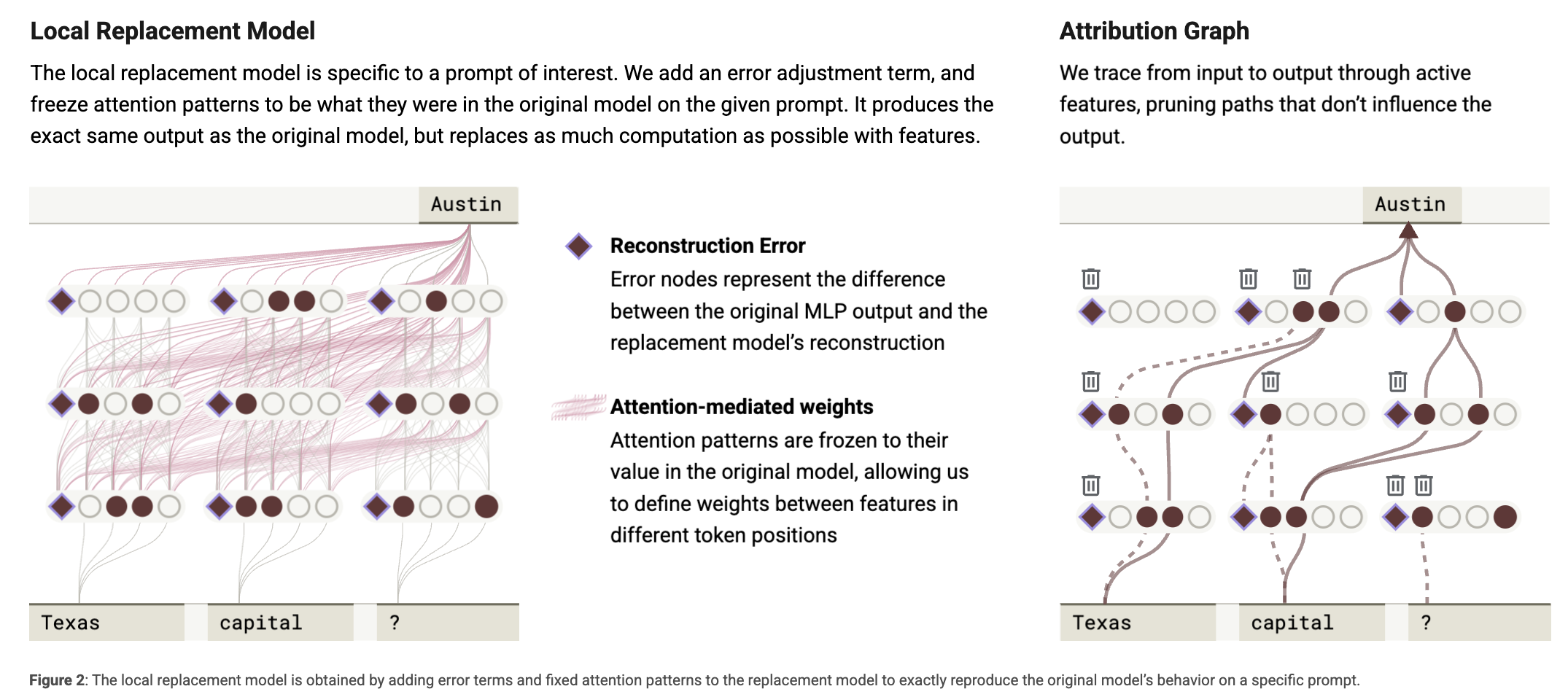
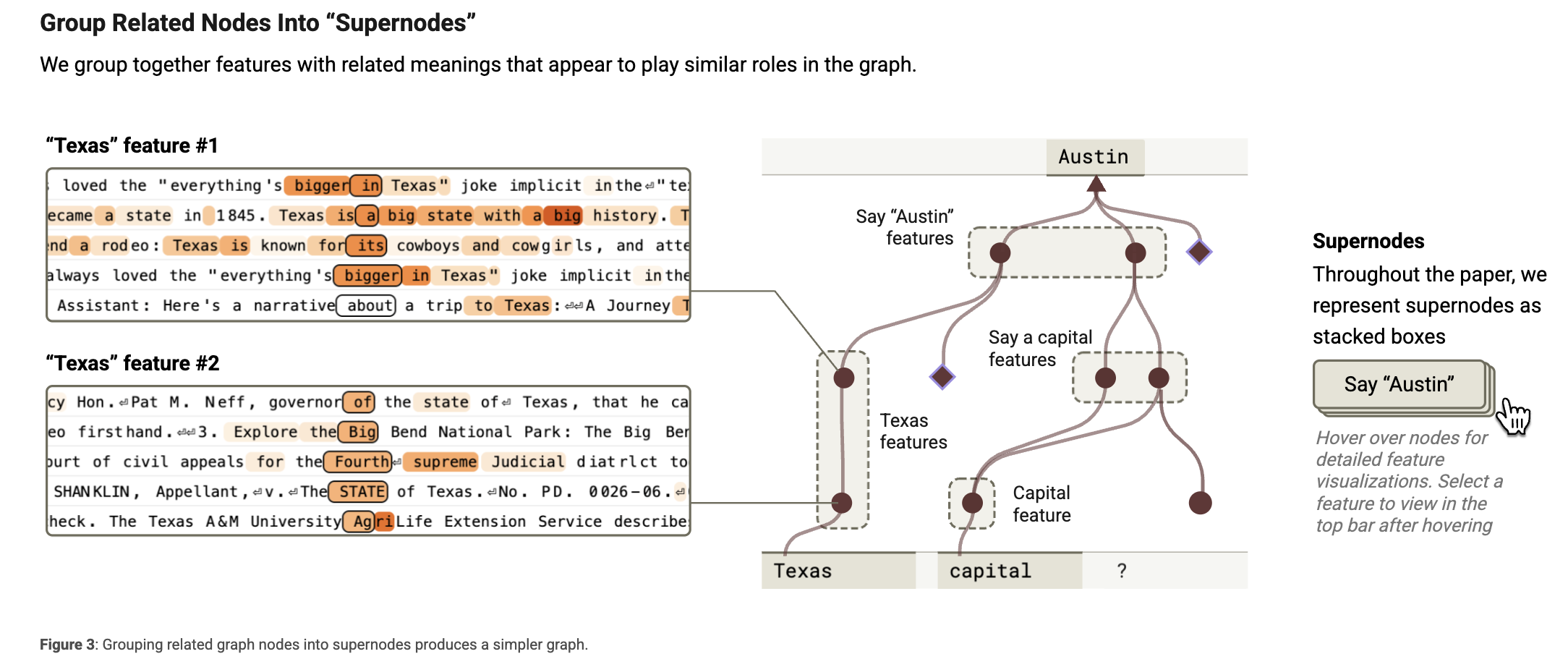
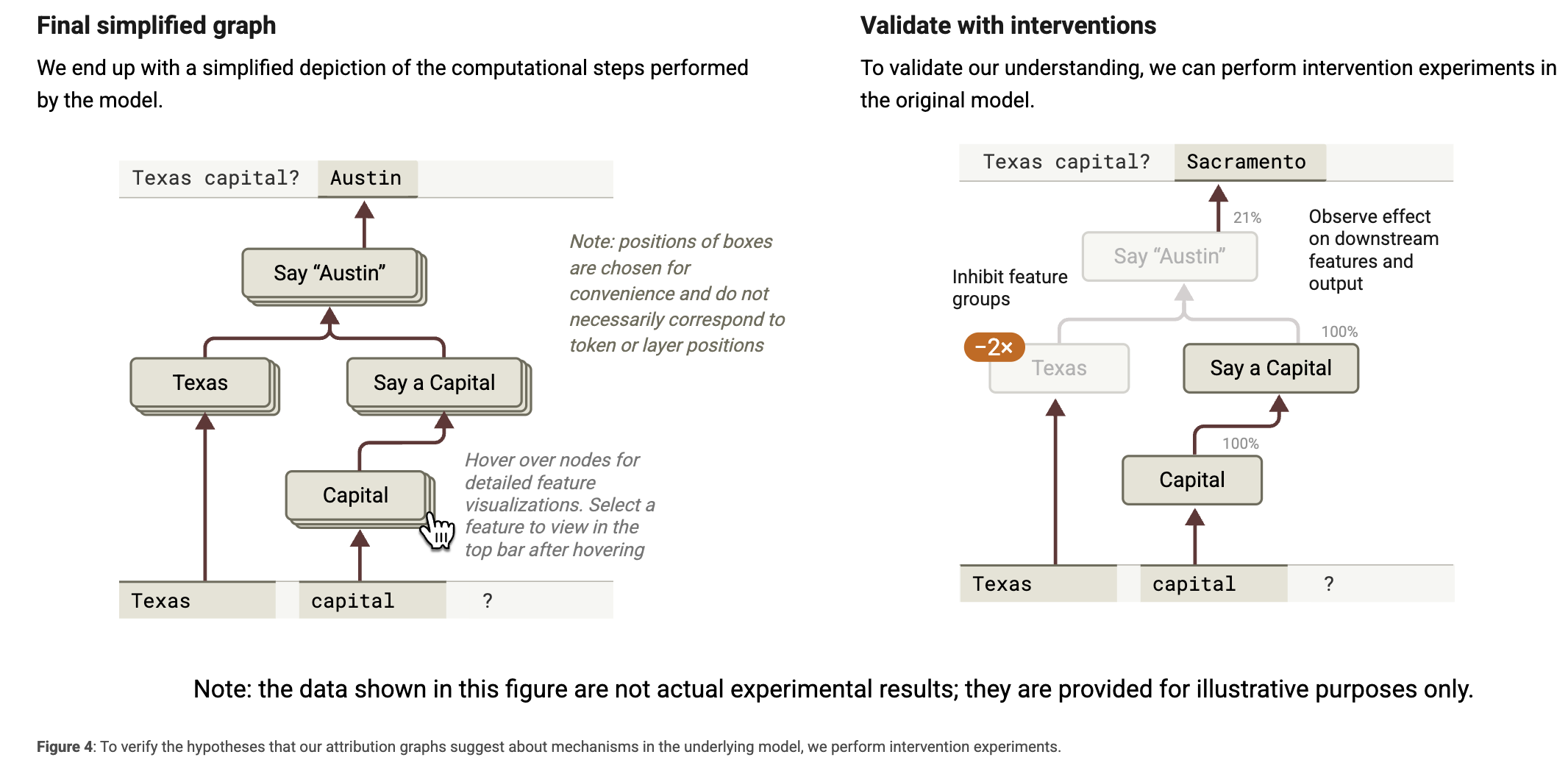
“Work Related to Chain-of-thought Faithfulness”
"Prior work has demonstrated that models’ chain-of-thought can be unfaithful, in the sense that the reasoning steps the model writes down are not causally related to its final answer[92, 36]. In these works, unfaithfulness is demonstrated by performing experiments that (a) modify an aspect of the prompt, observe a change in the model’s behavior, but observe no reference in the chain-of-thought to the aspect of the prompt that was modified, or (b) modify the content of the chain-of-thought (putting “words in the model’s mouth”) and observing its effects on the model’s final answer. In this work, by contrast, we attempt to distinguish faithful vs. unfaithful reasoning mechanistically, analyzing the model’s activations on a single prompt (and then validating our findings using a prompting experiment as above). Other recent work has also shown that the likelihood of unfaithfulness can be decreased by breaking down a question into simpler subquestions [93]. Our example may be related to this – the model resorts to unfaithful reasoning when the question it is asked is too hard for it to plausibly answer.”
https://transformer-circuits.pub/2025/attribution-graphs/biology.html
Entrepreneurs as theorists: on the origins of collective beliefs and novel strategies
What if what if?
“The process of entrepreneurial theorizing consists of three key conceptual elements: (1) the triggering role of experiential and observational fragments; (2) the imagination of possibilities; and (3) the process of reasoning and justification.”
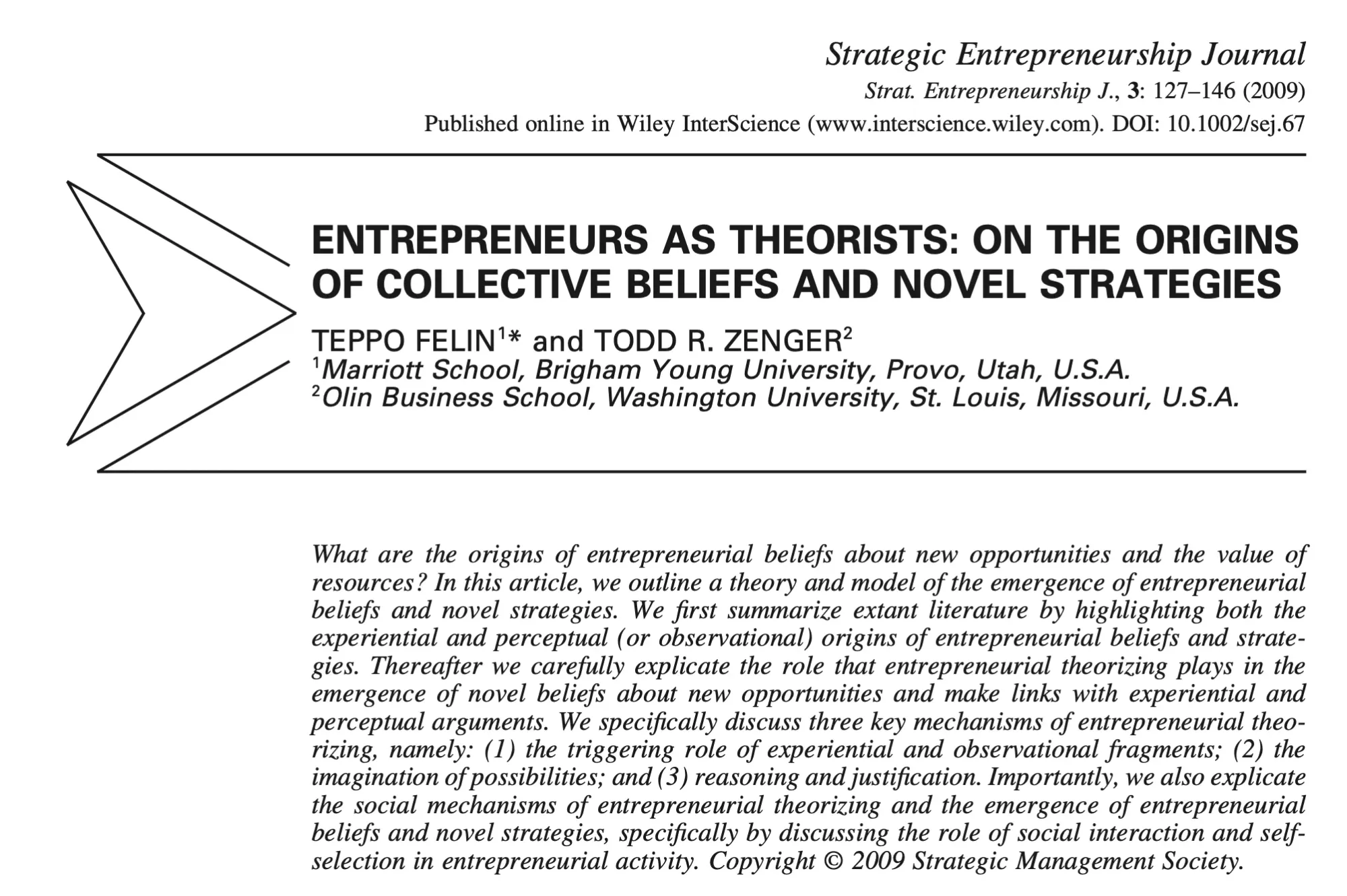
“In other words, the mechanisms of social interaction and self-selection not only shape entrepreneurial ideas and theories themselves, but also, importantly, provide the boundaries for which entrepreneurial theories are tested (specifically given that the testing of entrepreneurial theories requires sufficient collective buy in). In all, we argue that entrepreneurial theorizing provides a key organizational initial condition that importantly shapes subsequent organizational experience, search and learning, and more generally, the path-dependent future of organizations.”
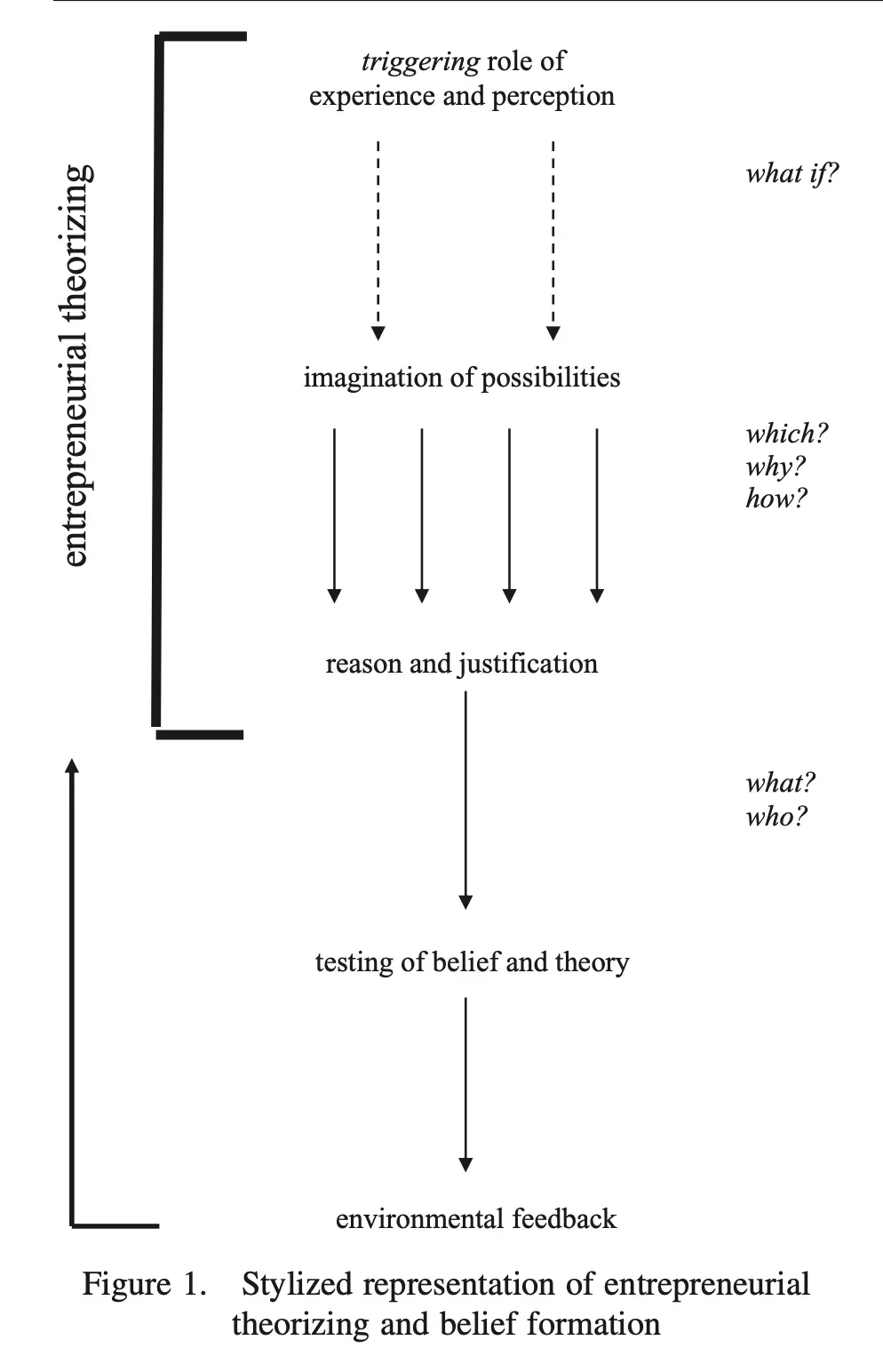
Felin, T., & Zenger, T. R. (2009). Entrepreneurs as theorists: on the origins of collective beliefs and novel strategies. Strategic Entrepreneurship Journal, 3(2), 127-146.
A grammar of hypotheses for visualization, data, and analysis
The assumption itself is interesting…
“Definition 1 (A Hypothesis). A hypothesis statement (h) is said to be in a hypothesis space H1 (i.e., h ∈ H1*) if h can be parsed by the grammar associated with the hypothesis space.”*
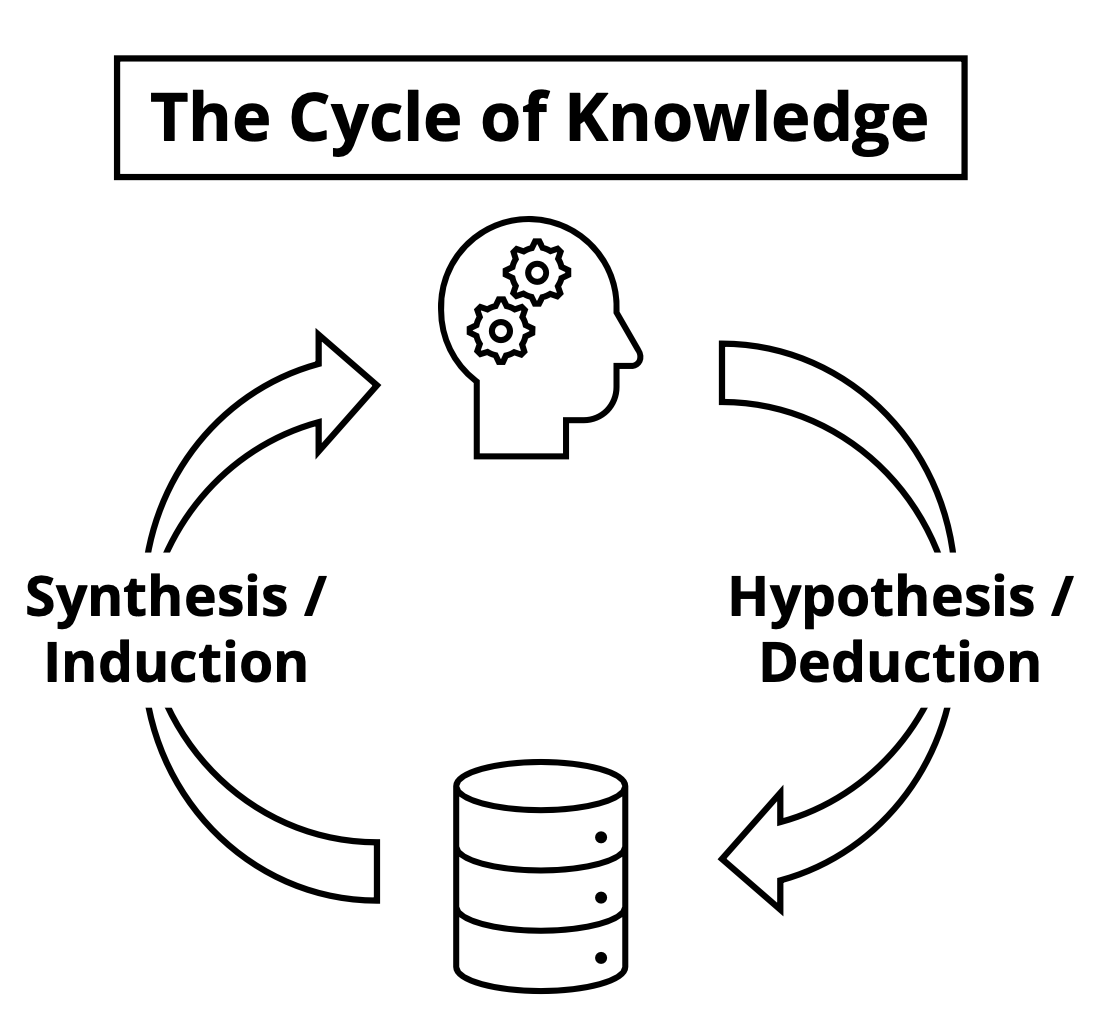
“A single hypothesis grammar cannot possibly operationalize all definitions of “tasks” in visualization. Our grammar is constrained to expressing analysis questions (as testable relationships between data variables) in the space of (tabular) data, and (mostly static) visual encodings. For example, our grammar can not encode tasks like “Enjoy [7],” as these tasks are not analytical questions in nature.
With this said, we believe a grammar-based approach to make analysis tasks operational is paramount.”
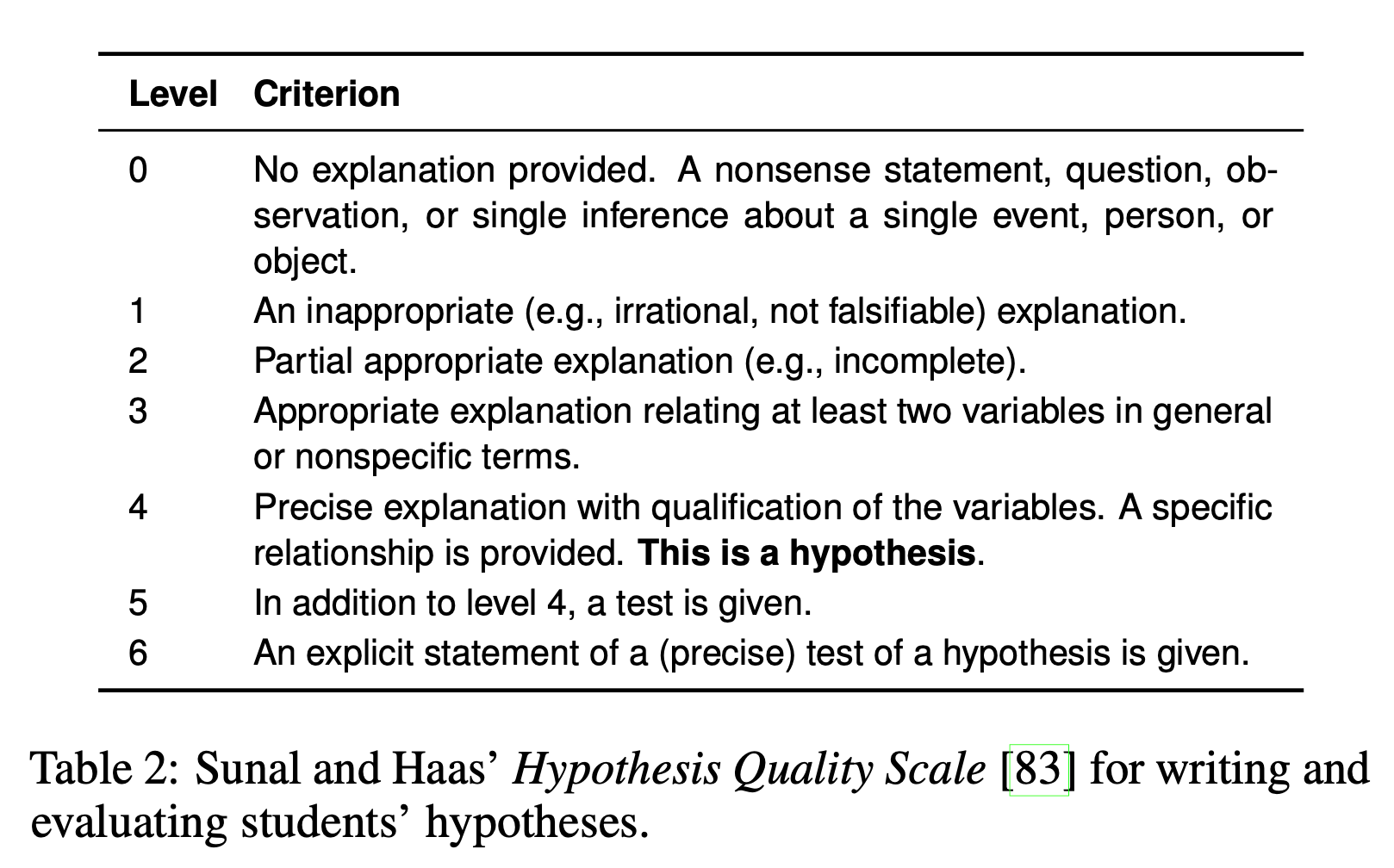
“This paper presented a grammar-based approach for operationalizing analysis tasks in visualization. The grammar draws from the science and education literature, and provides a formal language to express (scientific) hypotheses over datasets. We showed how sets of hypotheses (parsable by our grammar) make up a hypothesis space, and can be used to represent the hypotheses a specific dataset can evaluate (data hypothesis space), the hypotheses a visualization can evaluate (visualization hypothesis space), and the hypotheses a user wants to evaluate through analysis (analysis hypothesis space).
We illustrated how the use of our grammar can unify a user’s analysis goals, the available data, and the capabilities of a visualization system. We demonstrated how it can allow partial specification, and how it can formalize analysis tasks commonly used in visualization research.”
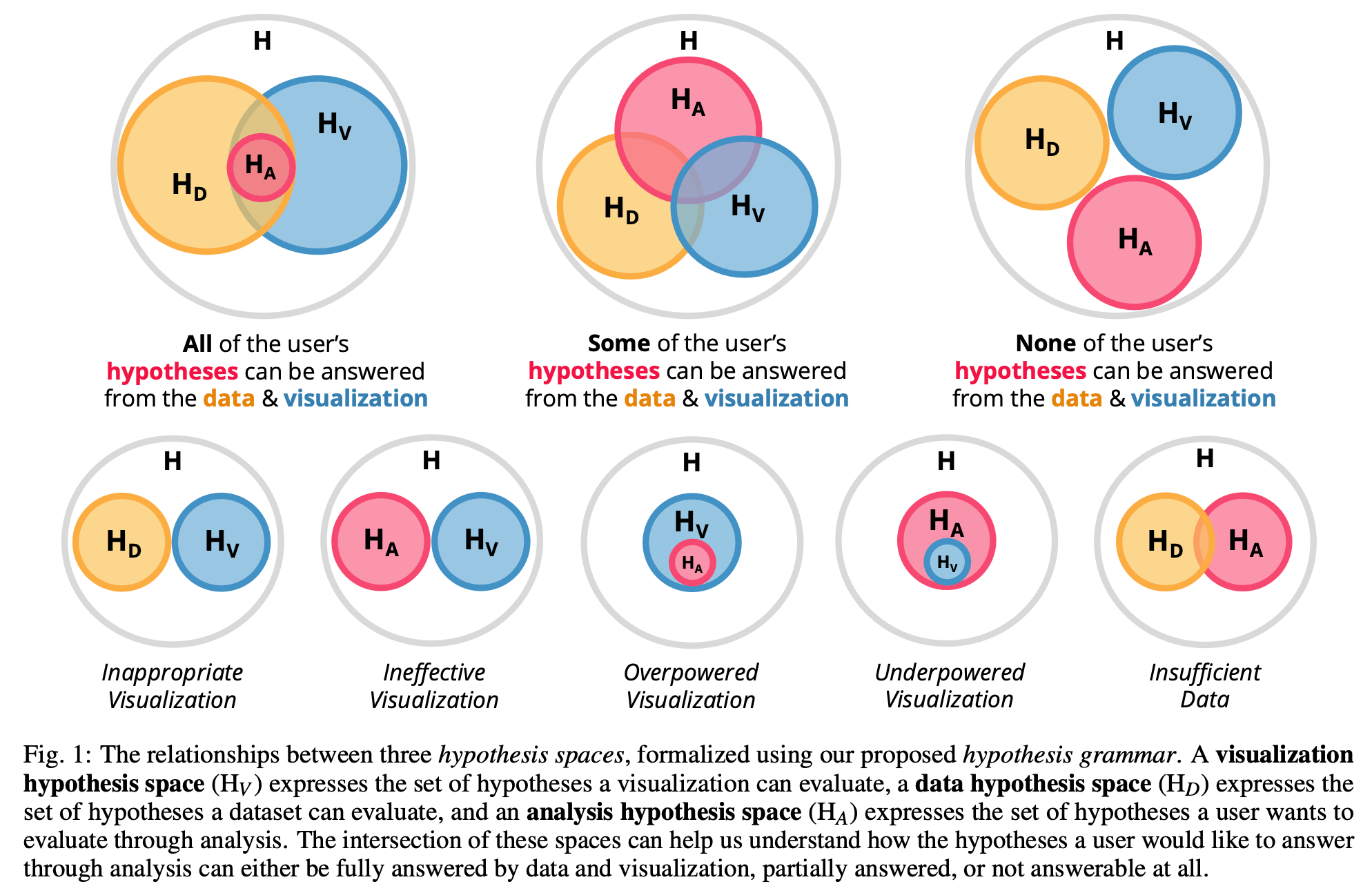
Suh, A., Mosca, A., Wu, E., & Chang, R. (2022). A grammar of hypotheses for visualization, data, and analysis. arXiv preprint arXiv:2204.14267.
https://arxiv.org/abs/2204.14267
Reader Feedback
“This stood out →‘Systemic stability is a classic public good’ — are you predicting more instability because of more agents in the wild?”
Footnotes
Some very interesting positioning from Bashy. And clean, fun, friendly branding.
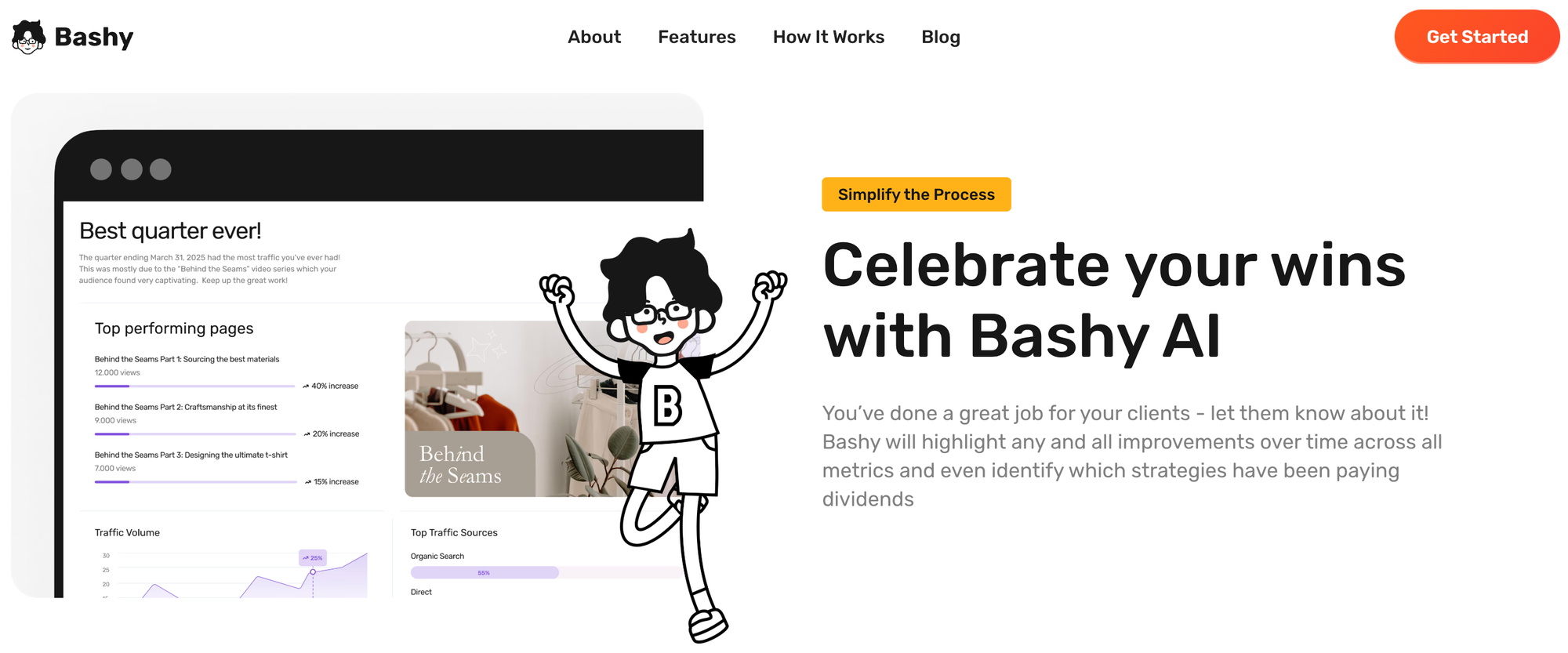
And if you're in Toronto this week:
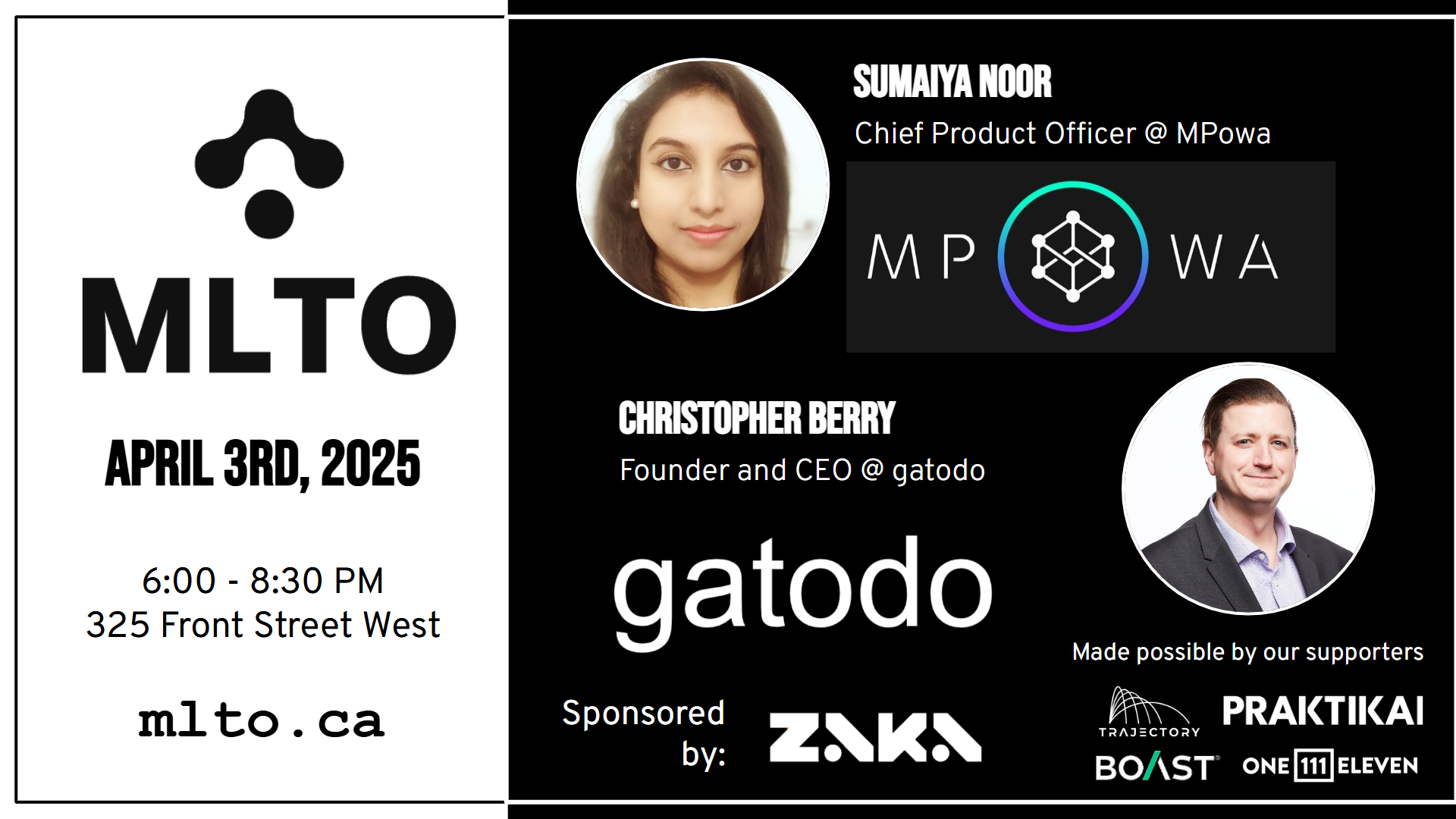
https://www.meetup.com/machine-learning-to-meetup/events/306723811/
Never miss a single issue
Be the first to know. Subscribe now to get the gatodo newsletter delivered straight to your inbox