If we tell them to maximize profit, they will
This week: Economic agents, cost of complexity, complexity comes and goes
Risks of AI as Economic Agents
If we tell them to maximize profit, they will.
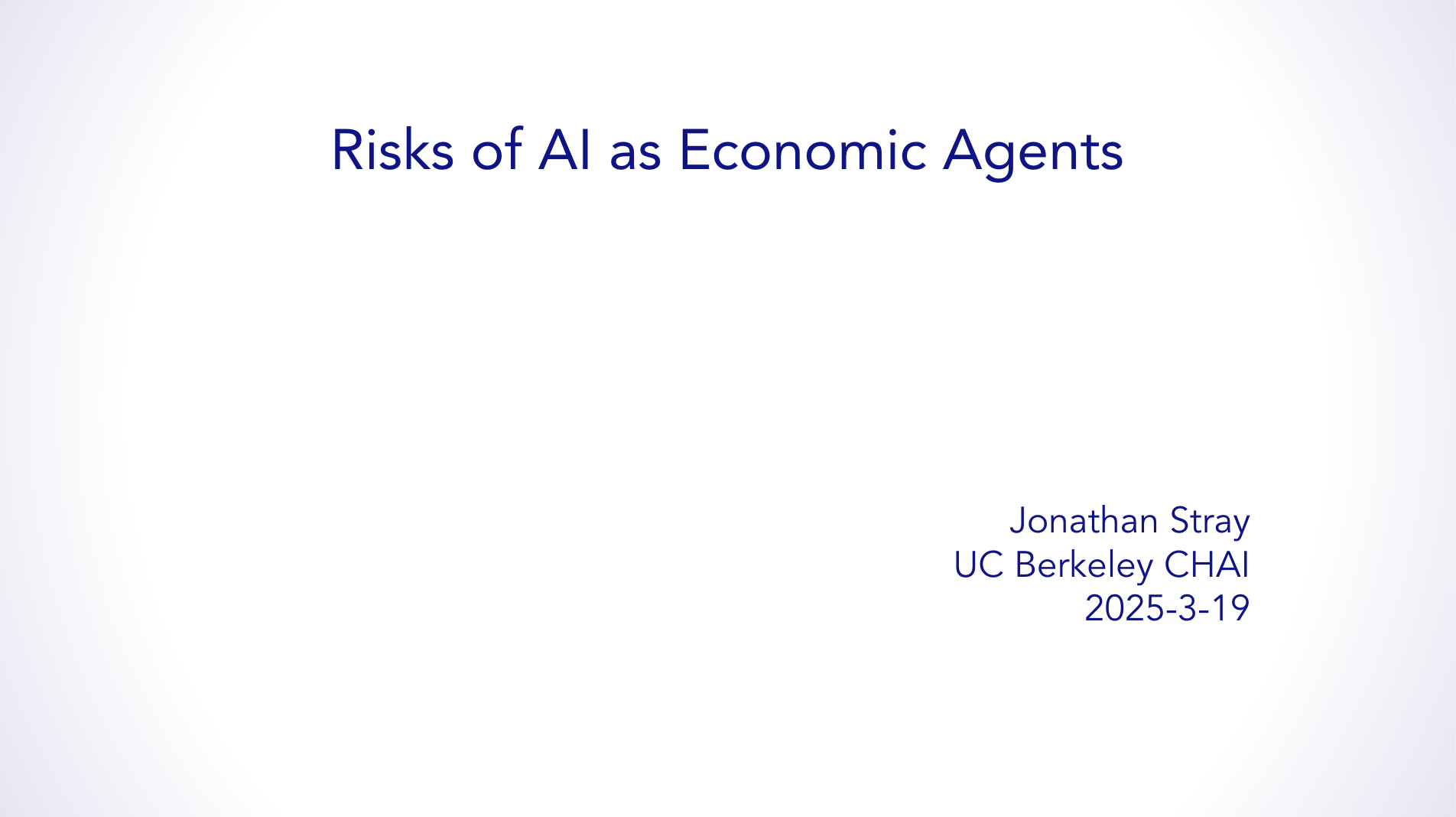
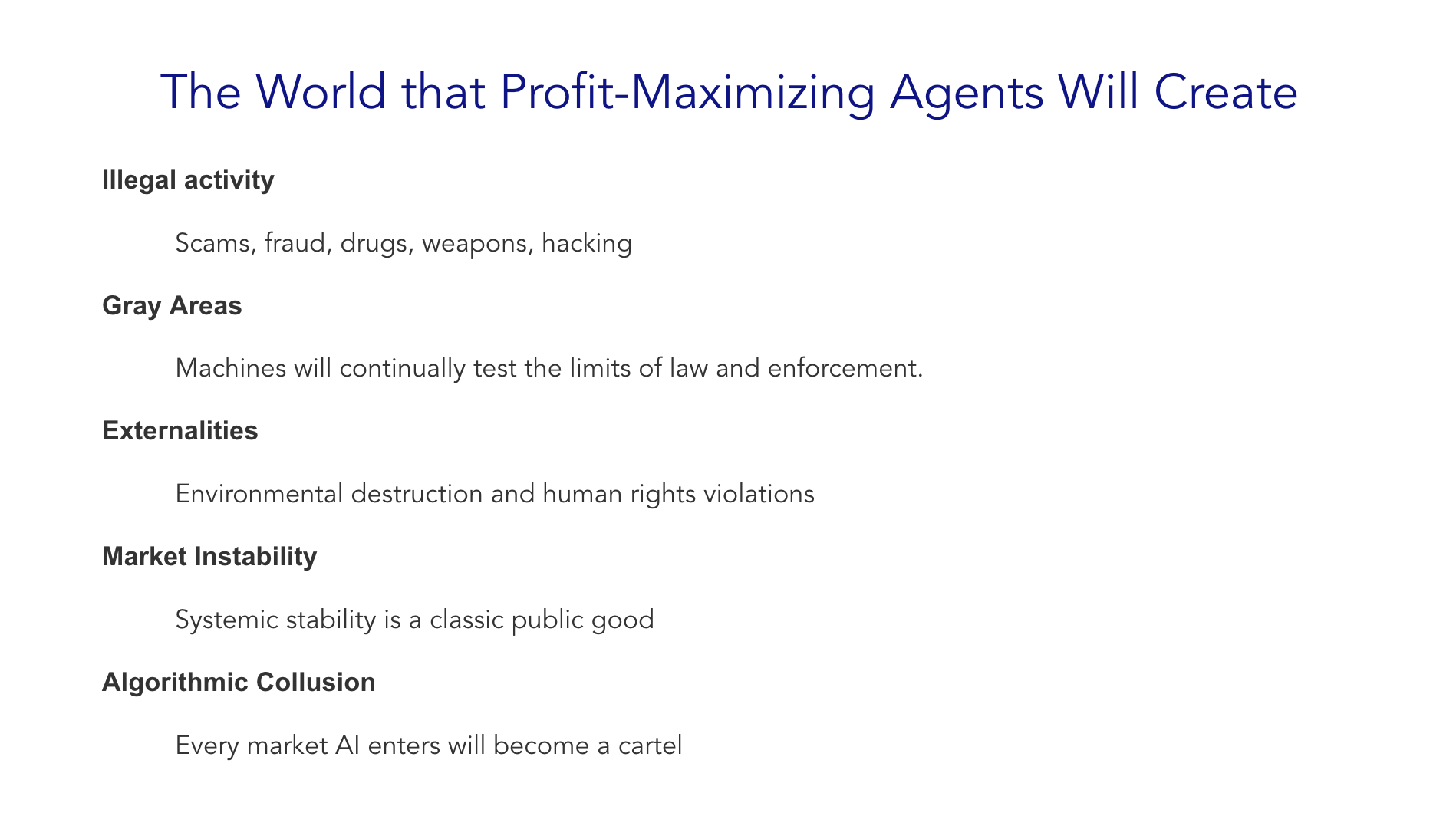
https://docs.google.com/presentation/d/1VqWP-MFnzp1mEnxdKohmVMMDrrSzd15-VBAfPVE6W2U/
https://bsky.app/profile/jonathanstray.bsky.social/post/3lkr4m5wxzc2a
Neurometabolic signaling and control of policy complexity
How does the brain regulate its investment of cognitive capacity?
“Complexity, quantified in bits, is metabolically expensive; it costs approximately 10^4 ATP molecules to transmit one bit of information at a chemical synapse 17, 27, 28”
“Beyond understanding the brain, this has implications for the design of AI systems, since it could inspire novel algorithms combining high-level resource monitors (analogous to HONs) with lower-level modules for cost-benefit analysis (akin to e.g. striatal dopamine systems), enabling systems to explicitly weigh the benefits of implementing complex behavioral policies against their related computational or energetic costs.”
“Crucially, this line of work emphasizes that agents often face a trade-o between the complexity of their decision policies and the computational or metabolic costs of implementing them. While empirical work and computational models appear to support the idea that our behavior approximates optimal policy compression under resource limitations, the neural mechanisms by which the brain signals and controls the complexity of behavioral policies remain poorly understood. Bridging this gap may thus require a tight integration of empirical neuroscience with computational frameworks to better understand the interplay between neural dynamics, environmental complexity, and adaptive behavior.”
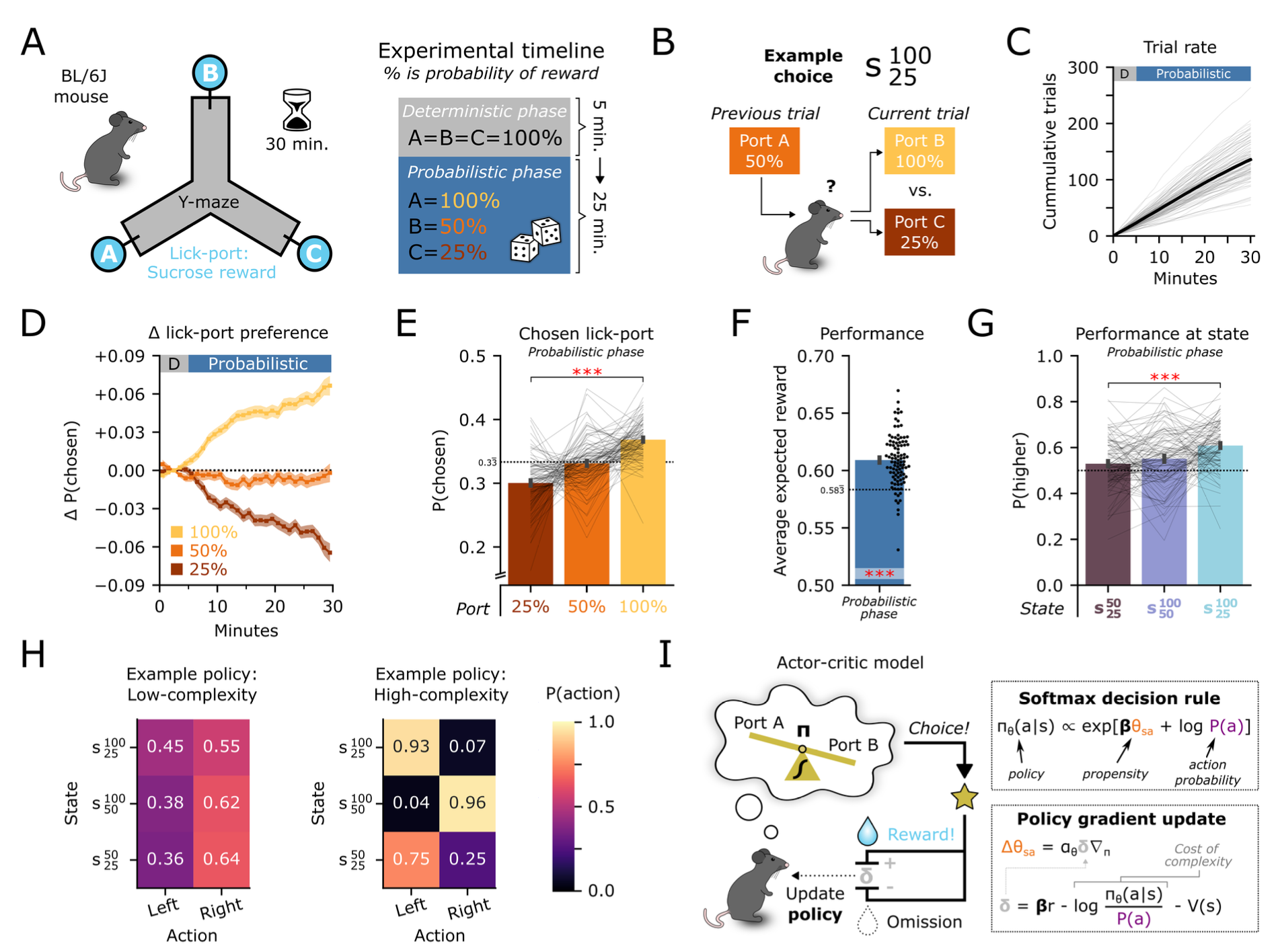
“…we implemented a multiarmed bandit task allowing us to examine the probabilistic relationships between actions, rewards, and states. We placed n = 97 BL/6J mice (n = 42 male and n = 55 female) into a 3-arm Y-Maze where each equidistant arm contained a lick- activated port distributing (probabilistic) sucrose reward (Fig. 1A, see methods). Each session consisted of two phases: a 5 minutes deterministic phase, serving as an adaptation period, in which reward probability was always 100%, and then a 25 minutes probabilistic phase where each port was pseudo-randomly assigned reward probability 25%, 50%, or 100%. Critically, a reward could not be dispensed from the same port twice in a row, thus creating three unique states 𝑠 for the task. In each state, mice had to implement an action 𝑎 indicating a left port or right decision. For example, in state 𝑠#$$ mice can only choose between the 100% and 25% probability !" port after the 50% port was selected in the previous trial (Fig. B). In each session mice continuously performed trials resulting in an average of 131 ± 4 trials across mice (Fig. 1C). Mice learned to choose the 100% port and were less likely to choose the 25% port (rmANOVA P<0.001, Fig. 1D-E) and the expected reward in the probabilistic phase (60.9% ± 0.3%) was better than chance (P<0.001, Fig. 1F), suggesting mice learned the task contingencies. Finally, we found performance was highest in state 𝑠#$$, while states 𝑠#$$ and 𝑠"$ proved more diIicult, indicating !" "$!" task performance was state-dependent (rmANOVA P<0.001, Fig. 1G). Broadly, we found minimal sex diIerences, although males tended to perform slightly more trials per session (Fig. S1).
“Our theory suggests that HONs may be seated at the top of a hierarchical structure governing resource-dependent behavioral policies, given that they are well positioned to sense metabolic signals but also innervate brain areas that guide reward processing and learning updates while considering policy complexity costs85 (striatum, VTA), and action selection (basal ganglia). While other systems may take care of implementing cost-benefit analyses30 lower in the “hierarchy tree”, a missing piece in the puzzle has been how the brain signals the resource availability to control resource investment in the first place. This study places HONs as a critical missing link providing a more comprehensive mechanistic model of strategic learning accounting for the interplay between decision-making policies and metabolic/informational constraints.”
Tesmer, A. L., Pola, C. D., Gilli, D., Grujic, N., Bracey, E., Patriarchi, T., ... & Burdakov, D. (2025). Neurometabolic signaling and control of policy complexity. bioRxiv, 2025-02.
https://www.biorxiv.org/content/10.1101/2025.02.24.639890v1.full.pdf
https://bsky.app/profile/alexandertesmer.bsky.social
15th Annual Biard Lecture - Sean Carroll "Complexity in the Universe"
“Entropy increases. Complexity comes and goes.”
“Physics has traditionally focused on the simplest possible systems — spherical cows.”
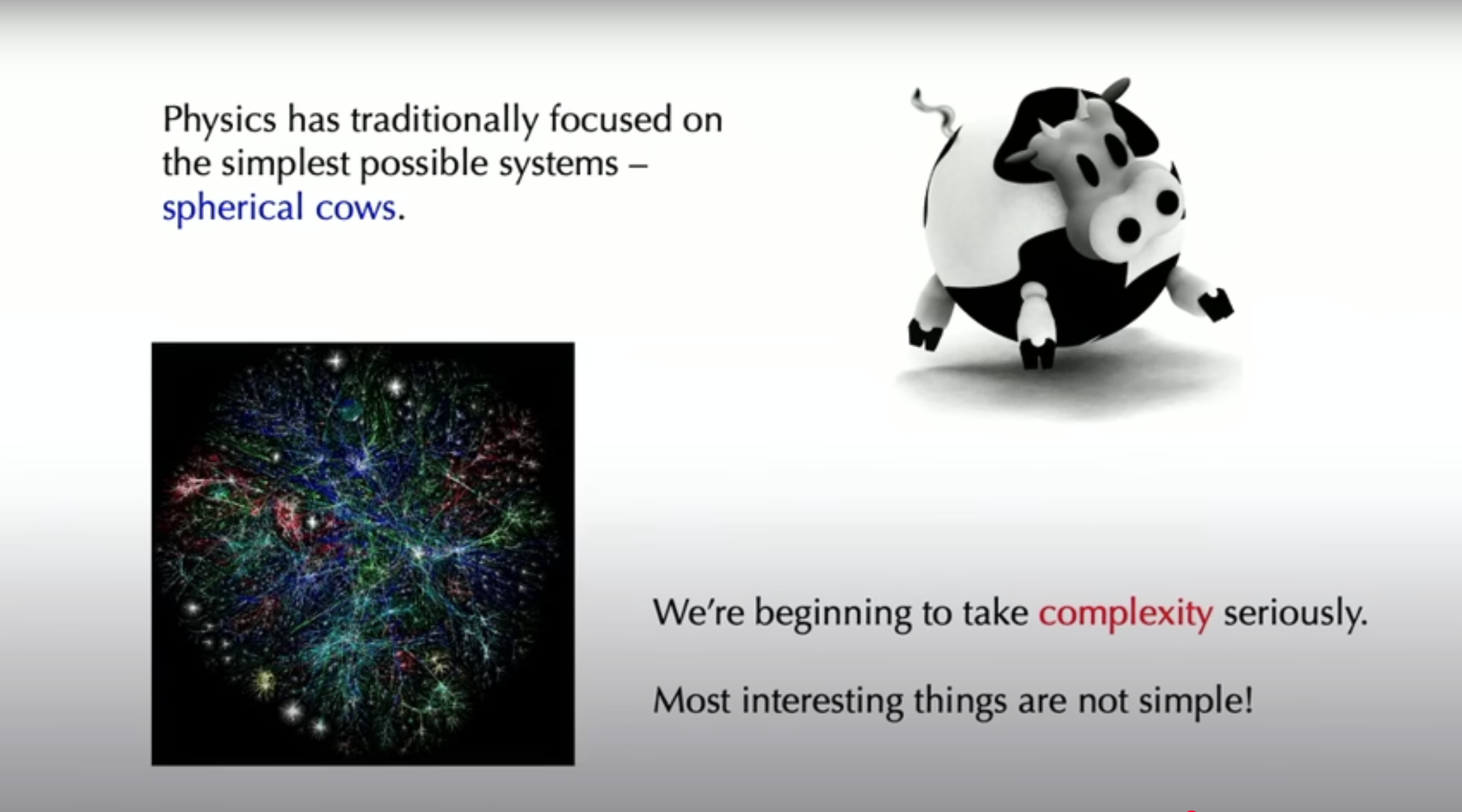
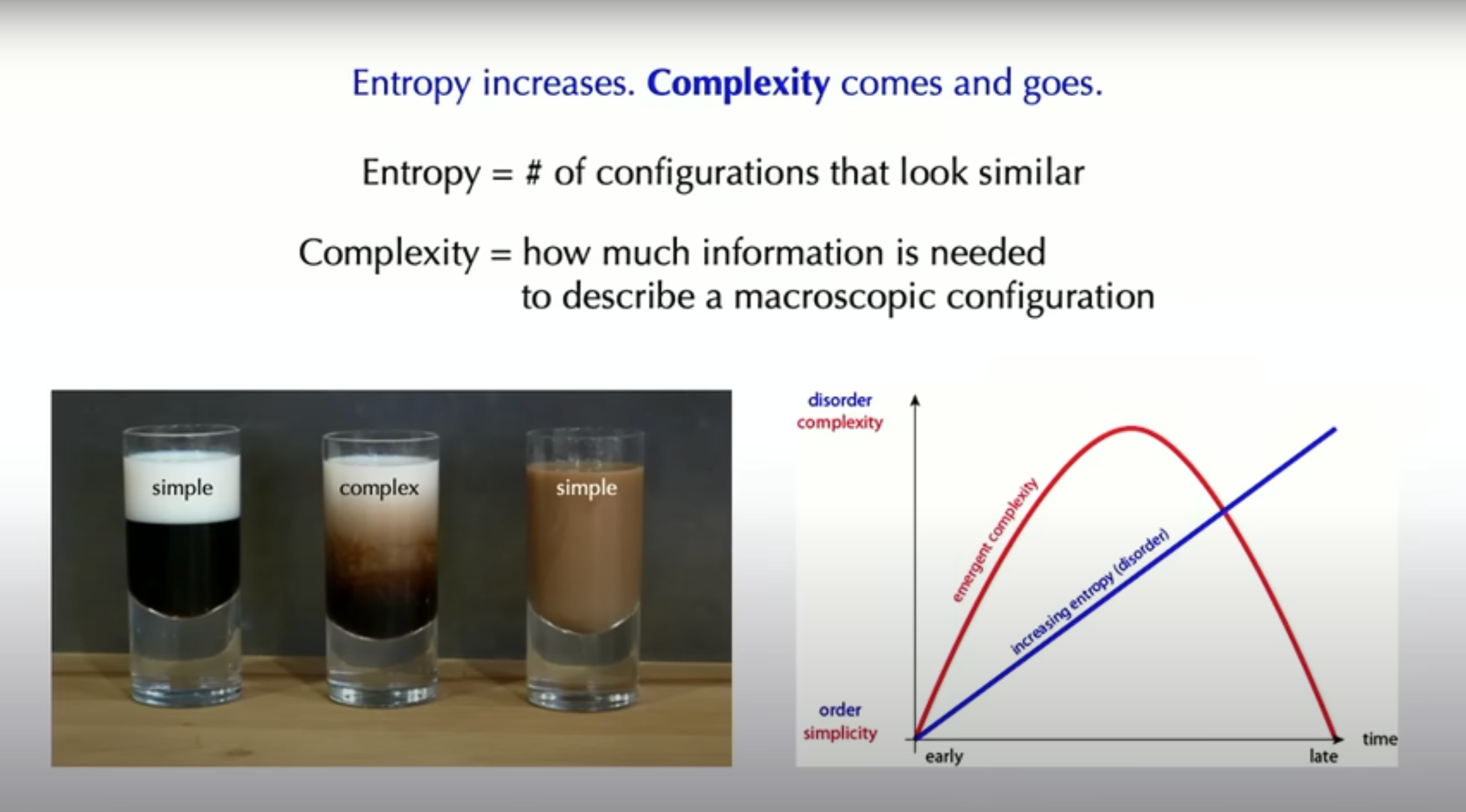
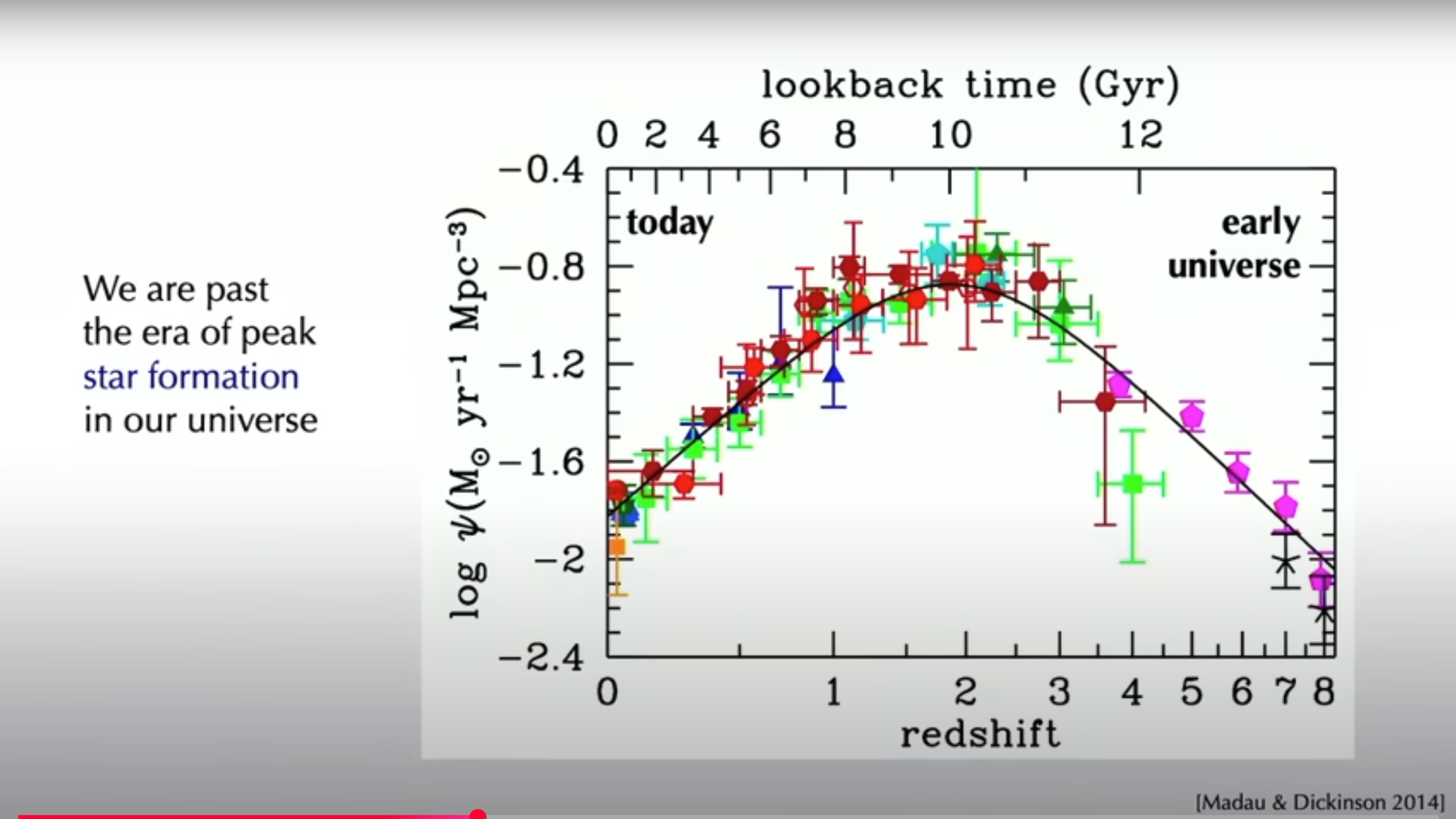
https://www.youtube.com/watch?v=Kr_S-vXdu_I
Reader Feedback
“It’s so much worse than eternal September.”
Footnotes
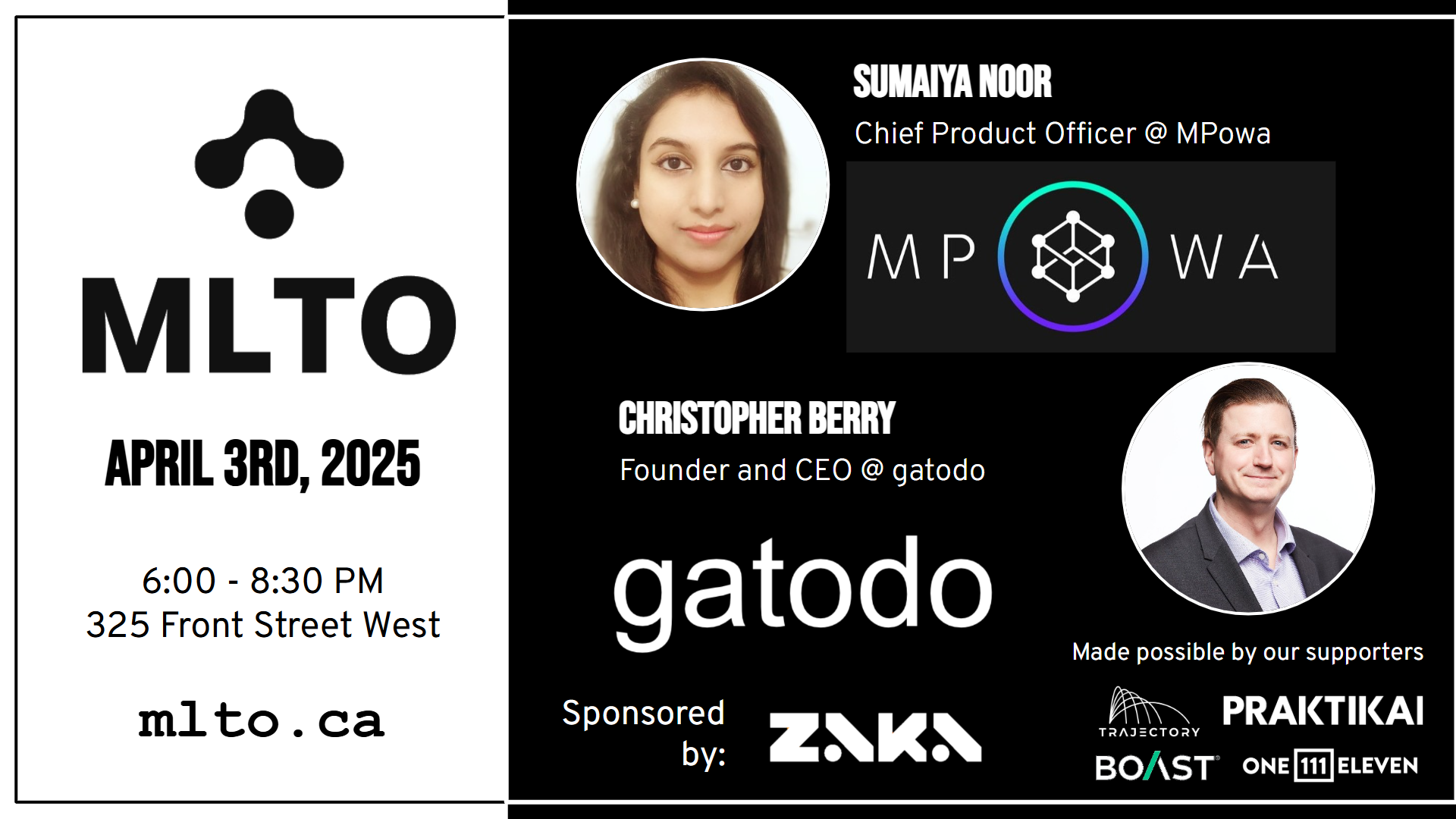
https://www.meetup.com/machine-learning-to-meetup/events/306723811/
Never miss a single issue
Be the first to know. Subscribe now to get the gatodo newsletter delivered straight to your inbox